Test-Time Optimization for Video Depth Estimation Using Pseudo Reference Depth
Computer Graphics Forum (Proceedings of Eurographics 2023)
-
Libing Zeng
Texas A&M University -
Nima Khademi Kalantari
Texas A&M University
Abstract
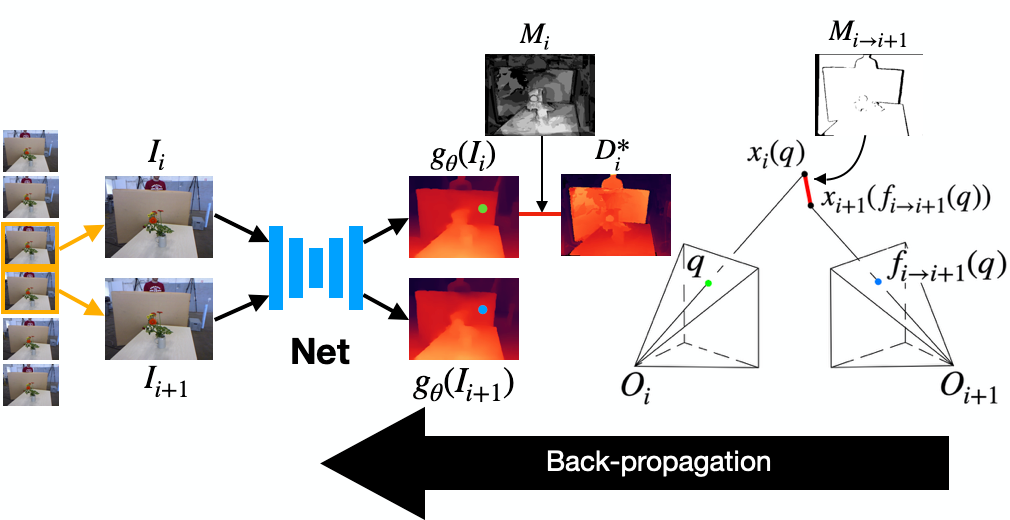
In this paper, we propose a learning-based test-time optimization approach for reconstructing geometrically consistent depth maps from a monocular video. Specifically, we optimize an existing single image depth estimation network on the test example at hand. We do so by introducing pseudo reference depth maps which are computed based on the observation that the optical flow displacement for an image pair should be consistent with the displacement obtained by depth-reprojection. Additionally, we discard inaccurate pseudo reference depth maps using a simple median strategy and propose a way to compute a confidence map for the reference depth. We use our pseudo reference depth and the confidence map to formulate a loss function for performing the test-time optimization in an efficient and effective manner. We compare our approach against the state-of-the-art methods on various scenes both visually and numerically. Our approach is on average 2.5× faster than the state of the art and produces depth maps with higher quality.
Results
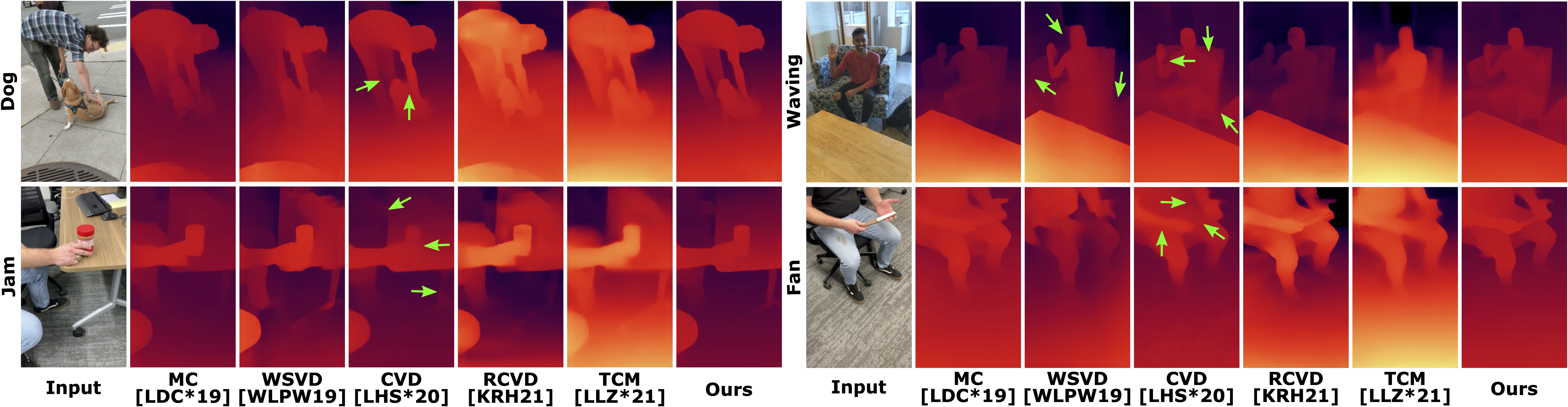
Comparisons against several state-of-the-art methods on casually captured dynamic scenes.
Eurographics 2023 Fast Forward
Eurographics 2023 Talk
Supplementary Video
BibTeX
@article{Zeng_2023_depth, author = {Zeng, Libing and Kalantari, Nima Khademi}, title = {Test-Time Optimization for Video Depth Estimation Using Pseudo Reference Depth}, journal = {Computer Graphics Forum}, doi = {https://doi.org/10.1111/cgf.14729}, url = {https://onlinelibrary.wiley.com/doi/abs/10.1111/cgf.14729}, }
Acknowledgements
We thank the reviewers for their insightful comments. We also thank Brennen Taylor for capturing the input sequences. The website template was borrowed from Michael Gharbi.